KeynotesWorkshop
Keynote Speakers
Keynote 1: Modeling of Perceptual Speaker Embedding and Its Application to Speech and Speaker Recognition
Prof. Sadaoki Furui
Among various information conveyed by spoken utterances, linguistic information about meanings that the speaker wanted to express and individuality information about the speaker are most basic and important for human communication. The human brain stores models of both information, and people recognize these two classes of information easily, clearly and simultaneously. People have common sense about the human voice, and using the common sense, people can capture the characteristics of each speaker's voice from extremely short utterance by each speaker and predict his/her voice uttering new words or sentences. Using this skill, people can separate the voices of many speakers spoken simultaneously or sequentially, and the contents of each utterance can be understood. Although various researches have been conducted on technologies for recognizing speakers of utterances, technologies for automatically adapting recognition models to speakers to improve speech recognition accuracy, and technologies for separating and extracting multiple superimposed utterances, their performances are far below human abilities. It is important to clarify the principle of speaker embedding, in which people model and use the personality of speech, and incorporate it into speech and speaker recognition systems in a semi-supervised or self-supervised manner.
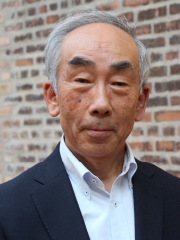
Sadaoki Furui received the B.S., M.S., and Ph.D. degrees from the University of Tokyo, Japan in 1968, 1970, and 1978, respectively. After joining the Nippon Telegraph and Telephone Corporation (NTT) Labs in 1970, he has worked on speech analysis, speech recognition, speaker recognition, speech synthesis, speech perception, and multimodal human-computer interaction. From 1978 to 1979, he was a visiting researcher at AT&T Bell Laboratories, Murray Hill, New Jersey. He was a Research Fellow and the Director of Furui Research Laboratory at NTT Labs. He became a Professor at Tokyo Institute of Technology in 1997. He was Dean of Graduate School of Information Science and Engineering, and Director of University Library. He was given the title of Professor Emeritus and became Professor at Academy for Global Leadership in 2011. He has served as President of Toyota Technological Institute at Chicago (TTIC) from 2013 to 2019, and is now serving as its Chair of the Board of Trustees. He has authored or coauthored over 1,000 published papers and books. He was elected a Fellow of the IEEE, the Acoustical Society of America (ASA), the Institute of Electronics, Information and Communication Engineers of Japan (IEICE) and the International Speech Communication Association (ISCA). He received the Paper Award and the Achievement Award from the IEEE SP Society, the IEICE, and the Acoustical Society of Japan (ASJ). He received the ISCA Medal for Scientific Achievement, and the IEEE James L. Flanagan Speech and Audio Processing Award. He received the NHK (Japan Broadcasting Corporation) Broadcast Cultural Award and the Okawa Prize. He also received the Achievement Award from the Minister of Science and Technology and the Minister of Education, Japan, and the Purple Ribbon Medal from Japanese Emperor. He was accredited as Person of Cultural Merit by the Japanese Government in 2016.
Keynote 2: Towards Unsupervised Learning of Speech Representations
Dr. Mirco Ravanelli
The success of deep learning techniques strongly depends on the quality of the representations that are automatically discovered from data. These representations should capture intermediate concepts, features, or latent variables, and are commonly learned in a supervised way using large annotated corpora. Even though this is still the dominant paradigm, some crucial limitations arise. Collecting large amounts of annotated examples, for instance, is very costly and time-consuming. Moreover, supervised representations are likely to be biased toward the considered problem, possibly limiting their exportability to other problems and applications. A natural way to mitigate these issues is unsupervised learning. Unsupervised learning attempts to extract knowledge from unlabeled data, and can potentially discover representations that capture the underlying structure of such data. This modality, sometimes referred to as self-supervised learning, is gaining popularity within the computer vision community, while its application on high-dimensional and long temporal sequences like speech still remains challenging.
In this keynote, I will summarize some recent efforts to learn general, robust, and transferrable speech representations using unsupervised/self-supervised approaches. In particular, I will focus on a novel technique called Local Info Max (LIM),that learns speech representations using a maximum mutual information approach. I will then introduce the recently-proposed problem-agnostic speech encoder (PASE) that is derived by jointly solving multiple self-supervised tasks. PASE is a first step towards a universal neural speech encoder and turned out to be useful for a large variety of applications such as speech recognition, speaker identification, and emotion recognition.
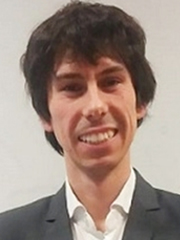
Mirco Ravanelli is currently a post-doc researcher at Mila (Université de Montréal) working under the supervision of Prof. Yoshua Bengio. His main research interests are deep learning, speech recognition, far-field speech recognition, robust acoustic scene analysis, cooperative learning, and unsupervised learning. He is the author or co-author of more than 40 papers on these research topics. He received his Ph.D. (with cum laude distinction) from the University of Trento in December 2017. During his Ph.D., he focused on deep learning for distant speech recognition, with a particular emphasis on noise-robust deep neural architectures.
Keynote 3: The importance of Calibration in Speaker Verification
Dr. Luciana Ferrer
Most modern speaker verification systems produce uncalibrated scores at their output. That is, while these scores contain valuable information to separate the same-speaker from the different-speaker trials, they cannot be interpreted in absolute terms, only relative to their distribution. A calibration stage is usually applied to the output of these systems to convert them into useful absolute measures that can be interpreted and reliably thresholded to make decisions. In this keynote, we will review the definition of calibration, present ways to measure it, discuss when and why we should care about it, and show different methods that can be used to fix calibration when necessary.
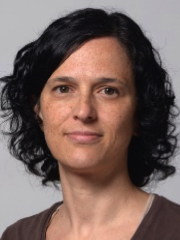
Luciana Ferrer is a researcher at the Computer Science Institute (ICC, for its acronym in Spanish), affiliated to the University of Buenos Aires (UBA) and the National Scientific and Technical Research Council (CONICET), Argentina. Luciana received her Ph.D. degree in Electronic Engineering from Stanford University, USA, in 2009, and her Electronic Engineering degree from the University of Buenos Aires, Argentina, in 2001. Her primary research focus is machine learning applied to speech processing tasks.